Harnessing AI for Safer Urban Infrastructure: Enhancing Liquefaction Resilience with Machine Learning Models
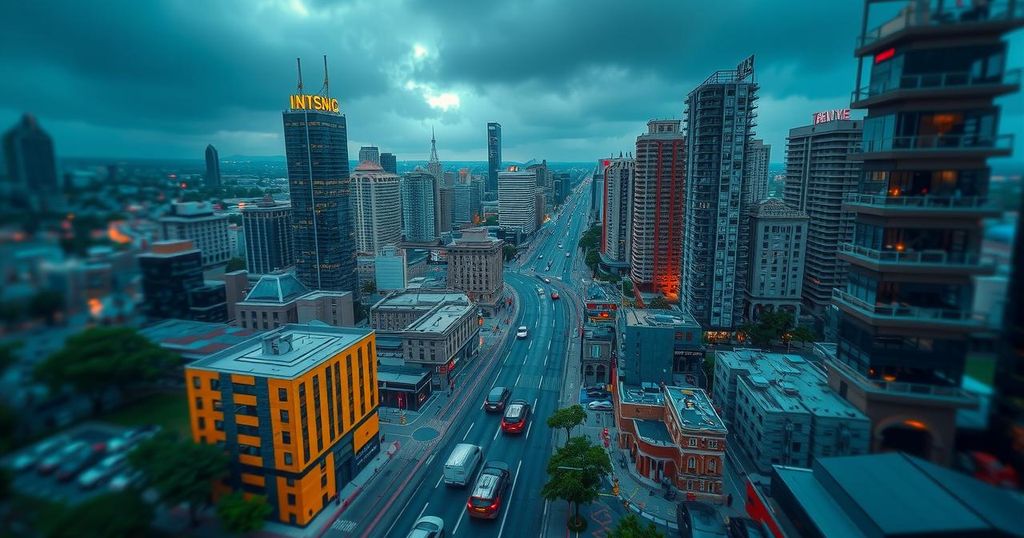
This article discusses the use of artificial intelligence and machine learning to develop predictive models that identify areas vulnerable to soil liquefaction in earthquake-prone cities like Tokyo. The research, conducted by Professor Shinya Inazumi and student Yuxin Cong, utilizes artificial neural networks and ensemble techniques to create accurate 3D soil maps. These advancements aid in identifying stable construction sites and enhancing urban resilience against seismic risks, thus contributing to safer city planning and disaster management strategies.
The application of artificial intelligence (AI) and machine learning in urban planning is significantly enhancing city resilience, particularly in identifying susceptibility to liquefaction in earthquake-prone regions like Japan. Researchers, led by Professor Shinya Inazumi and his student Yuxin Cong from Shibaura Institute of Technology, have developed advanced machine learning models that effectively predict soil stability by creating detailed three-dimensional maps of soil-bearing layers. By analyzing data from 433 test locations in Setagaya, Tokyo, these models empower city planners to determine areas at risk of liquefaction and strategically identify locations suitable for safe construction. This innovation directly addresses the dangers posed by soil liquefaction, a phenomenon where saturated, loose soil loses its stability during seismic events, thus endangering infrastructure and human life. The team employed artificial neural networks (ANNs) and bagging techniques to enhance prediction accuracy, resulting in a significant improvement in the reliability of soil assessments. This research emphasizes the importance of utilizing data-driven methodologies to fortify urban infrastructure against the devastating impacts of natural disasters, ultimately fostering safer urban environments.
The phenomenon of soil liquefaction is a critical concern for urban planners in seismically active regions, where natural disasters threaten public safety and infrastructure integrity. Liquefaction occurs when intense ground shaking from an earthquake causes saturated soil to behave like a liquid, leading to severe structural damage. Notable examples include the 2011 Tōhoku earthquake in Japan, which caused extensive liquefaction-related damage, and similar incidents in Christchurch and Noto. In light of these risks, the application of innovative technologies such as machine learning is emerging as a key strategy for improving urban resilience and disaster preparedness by allowing for thorough analysis and prediction of soil behavior during seismic activity.
In conclusion, the integration of artificial intelligence in urban planning signifies a transformative advancement in enhancing city resilience, particularly in areas vulnerable to liquefaction caused by earthquakes. The research conducted by Professors Inazumi and Cong illustrates the potential of machine learning to refine predictions of soil stability and inform safer construction practices. By utilizing comprehensive data analysis, cities can better mitigate risks associated with natural disasters, thereby promoting safer urban development and stronger infrastructure.
Original Source: www.preventionweb.net