Enhancing Urban Resilience Against Liquefaction: Machine Learning Innovations in Seismically Active Zones
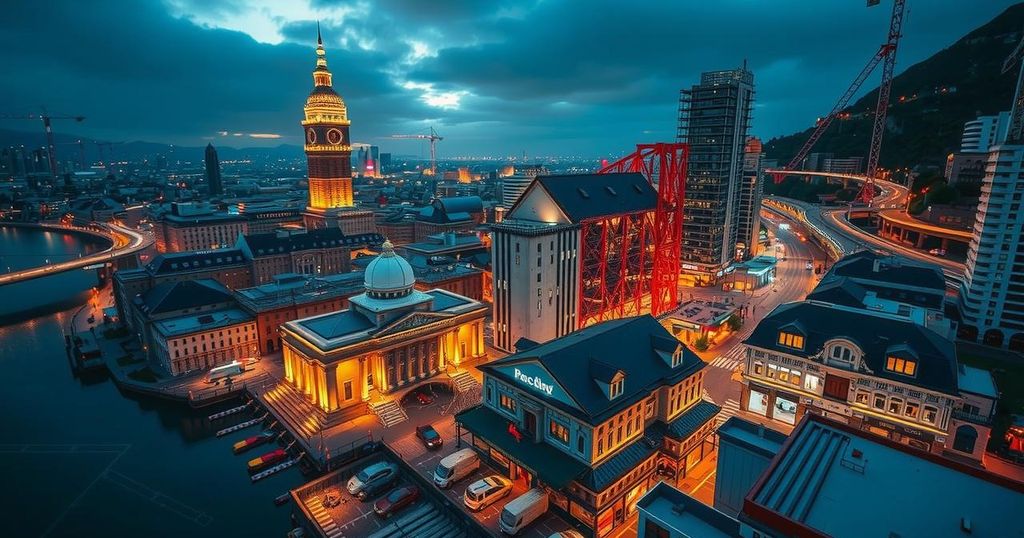
In Japan, the threat of soil liquefaction during earthquakes poses significant risks to infrastructure. Researchers from Shibaura Institute of Technology, led by Professor Shinya Inazumi, have developed machine learning models to predict soil stability during seismic events. By utilizing geological data and artificial neural networks, these models provide a more extensive understanding of potential construction sites, aiding civil engineers and disaster management professionals in their planning efforts. Their recent study published in “Smart Cities” outlines methods for improving urban resilience against liquefaction risks through data-driven strategies.
As urban development accelerates, the potential for natural disasters poses an increasing challenge for city planners and disaster response teams, particularly in seismically active regions such as Japan. A significant concern within this framework is soil liquefaction, a critical hazard that emerges during seismic events. This process occurs when intense ground shaking causes loose, saturated soils to behave like a liquid, leading to severe structural damage. Historical data underscores the peril associated with liquefaction, as evidenced by the devastation during notable earthquakes, including the Tōhoku earthquake in 2011, which resulted in liquefaction affecting 1,000 homes, and the 6.2 magnitude earthquake in Christchurch, where 80% of the water and sewage infrastructure was compromised. This reality was similarly echoed during the 2024 Noto earthquake, which resulted in widespread liquefaction impacting 6,700 residential properties. To bolster the resilience of urban infrastructure against such adversities, Professor Shinya Inazumi and his student, Yuxin Cong, from the Shibaura Institute of Technology are pioneering the application of machine learning techniques to accurately predict soil behavior during earthquakes. Their innovative approach involves leveraging geological data to devise comprehensive 3D models of subsurface soil layers, enabling the identification of areas that are structurally secure as opposed to those vulnerable to liquefaction. This predictive approach greatly surpasses the limitations of traditional manual soil assessments, which often struggle to cover extensive areas. Detailed findings of their research were published on October 8, 2024, in the journal “Smart Cities.” The study focuses on the utilization of artificial neural networks (ANNs) and ensemble learning methodologies to adeptly estimate the depth of bearing layers—an essential factor in determining soil stability during seismic activities. According to Professor Inazumi, “This study establishes a high-precision prediction method for unknown points and areas, demonstrating the significant potential of machine learning in geotechnical engineering. These improved prediction models facilitate safer and more efficient infrastructure planning, which is critical for earthquake-prone regions, ultimately contributing to the development of safer and smarter cities.” The research team utilized empirical data derived from 433 standard penetration tests and mini-ram sounding tests conducted in Setagaya-ku, Tokyo, to train their ANN model. This model enabled predictions of bearing layer depths at additional locations, with actual measurements assisting in validating the accuracy of the forecasts. The employment of a technique known as bagging (bootstrap aggregation) further maximized prediction precision, attaining a notable 20% improvement. The resulting contour maps reflect the estimated depths of stable soil layers within a one-kilometer radius around key sites, serving as critical resources for civil engineers. These visual aids are instrumental in identifying optimal construction zones and in assessing potential vulnerability to soil liquefaction, thus allowing for enhanced disaster risk management strategies. The researchers advocate for the integration of their method into the broader context of smart city planning, stressing the pivotal role of data-driven strategies in the stewardship of urban growth and infrastructure. Professor Inazumi notes, “This study provides a foundation for safer, more efficient, and cost-effective urban development. By integrating advanced AI models into geotechnical analysis, smart cities can better mitigate liquefaction risks and strengthen overall urban resilience.” Future work will aim to refine the model further by integrating additional geotechnical conditions and formulating specialized models for both coastal and inland regions, thereby accounting for groundwater influences—an essential aspect in liquefaction dynamics.
The increasing urbanization in seismically active areas, particularly in countries such as Japan, necessitates advanced measures to counteract natural disaster risks, especially the soil liquefaction phenomenon occurring during earthquakes. This risk significantly impacts infrastructure stability and public safety, compelling researchers and engineers to enhance predictive capabilities regarding soil behavior under seismic loads. The recent advancements using machine learning present promising avenues for more accurate assessments of ground stability, enabling urban planners to make informed decisions related to safe construction practices in disaster-prone regions.
The development of machine learning models by Professor Shinya Inazumi and Yuxin Cong represents a paradigm shift in addressing the risks associated with liquefaction in earthquake-prone regions. By providing detailed predictive analytics of soil behavior, these models are set to revolutionize urban planning and disaster management strategies, ultimately enhancing community resilience against seismic threats. This innovative approach not only addresses immediate infrastructural concerns but also contributes to the broader goals of developing safer and more sustainable urban environments.
Original Source: techxplore.com